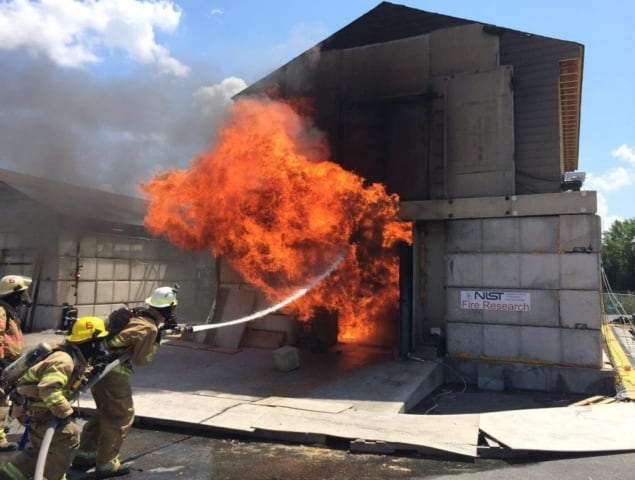
New machine learning algorithms could soon help firefighters forecast dangerous flashover ignition events using sensor data from burning buildings. Called P-Flash, the system was developed by Thomas Cleary and colleagues at the National Institute of Standards and Technology (NIST) in the US and Hong Kong Polytechnic University. Trained using data from thousands of simulated fires, the model can predict some flashovers in housefires up to 30 s before they occur.
Flashovers are among the most hazardous threats faced by firefighters. At high temperatures, all exposed combustible material in a room can be ignited simultaneously, releasing a huge amount of energy. To avoid danger, while maximizing the amount of time spent searching a fire for victims, it is critical for firefighters to predict these events as far in advance as possible. However, firefighters engaged in life-saving operations in smoky environments can sometimes overlook the characteristic precursors to flashovers such increasingly heat and flames rolling across the ceiling.
In their study, Cleary’s team aimed to develop more robust forecasting techniques based on the data gathered by heat sensors – which are often installed alongside smoke alarms in modern US homes. While these devices tend to fail above 150 °C, the team believes that prior to failure, data from the sensors can be used to measure temperature trends in rooms and track the distribution of heat throughout a building. From this information, machine learning algorithms could predict when and where flashovers will occur.
Virtual three-bedroom house
To demonstrate this, Cleary and colleagues developed the Prediction Model for Flashover (P-Flash), which they trained using data from more than 4000 simulated fires in a virtual three-bedroom house. In each simulation, they varied details including the arrangement of furniture in each room, and which windows and doors were open or closed. After training, the team then fine-tuned the model’s predictions using 500 additional simulations, then tested its performance with a final 500 runs. By capturing the complex relationship between temperature signals and flashover conditions, P-Flash predicted simulated flashover events 1 min in advance some 86% of the time.
Fighting fire with fire
Next, the researchers tested their model’s performance in 13 real housefires, carried out in controlled conditions at UL (formerly Underwriters Laboratories). When fires were started in open spaces like kitchens and living rooms, Cleary’s team found that P-Flash could successfully forecast a flashover 30 s before it occurred. However, the system was far less accurate at predicting flashovers in smaller rooms such as bedrooms.
The team now aims to tackle this shortcoming by further training of P-Flash with a focus on smaller rooms. If P-Flash can be improved, it could be installed on handheld devices that communicate with heat sensors via the cloud. This could help firefighters to predict the likely times and locations of flashovers before even arriving at the scene.
The researcher is described in Proceedings of the AAAI Conference on Artificial Intelligence.