
A new machine learning technique has significantly reduced noise in images taken by scanning transmission electron microscopy (STEM). Developed by researchers in Ireland, the algorithm could make it far easier for researchers to use weak electron beams to study sub-nanoscale details in delicate samples.
STEM is one of the most powerful tools available to researchers who study the atomic-scale structure and chemical composition of material samples. A focused electron beam is scanned across a thin sample and the electrons that pass through the sample are detected to create an image. Modern STEM techniques have now achieved imaging resolutions below 0.1 nm, so the technique is capable of seeing individual atoms.
However, achieving high resolutions comes at a cost. One is that higher resolutions are often achieved by using higher-intensity electron beams, which can damage or even destroy samples. A common example is knock-on damage, whereby an intense electron beam can shift the positions of atoms in a sample.
Damage or resolution
“Reducing the electron dose would limit the sample damage, but also the chance to extract useful information from the images, which will have worse resolution,” explains Laura Gambini of Trinity College Dublin, who led the research. “This is due to the presence of Poisson noise, the effect of which increases when reducing the number of incident electrons.”
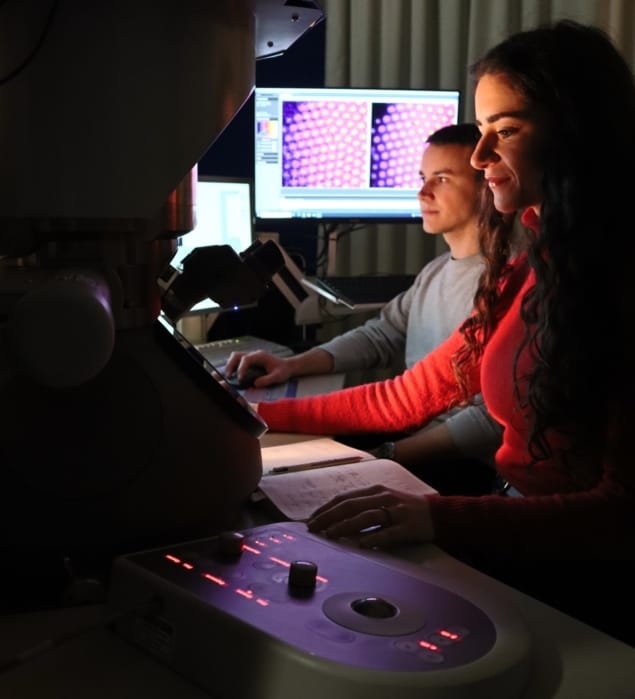
Since Poisson noise is a fundamental property of electron beams, it cannot simply be corrected by adjusting a STEM instrument, as is the case with some other types of noise. In their study, Gambini and her colleagues looked to machine learning algorithms, systems that learn to perform tasks by processing training data.
In this case, the team explored whether machine learning algorithms could be trained to reduce the noise in STEM images that have been scanned with low-dose electron beams. “Specifically, we trained an autoencoder on a dataset made of simulated STEM images, being careful in reflecting proper microscope settings and specimen variety in our dataset,” Gambini explains.
In particular, the researchers took care to ensure these data included a broad range of electron beam intensities, and that their training data were not biased towards samples with periodic crystal structures. After training their autoencoder, Gambini and colleagues tested its ability to reduce Poisson noise by using the system to process an extensive set of both real and artificial STEM images.
When they visually compared the images before and after processing, they noticed a clear reduction in noise. To quantify this improvement more precisely, they developed a benchmarking protocol based on atom localization – which estimates the positions of atoms within a sample.
Scanning transmission electron microscopy explained
By comparing this benchmarked data with their experimental results, the researchers demonstrated a greatly enhanced performance when using their autoencoder. “In a nutshell, we have demonstrated that our de-noised images contain more precise information than that in the original ones, and in those processed with other methods,” Gambini says.
Along with noise removal, the team’s approach offers a host of advantages that would make it easy to integrate with existing STEM techniques. “Our algorithm doesn’t require any human input or prior knowledge of the data, it can be implemented with no need to buy any additional expensive equipment for the microscope, and it can run at a speed compatible with live data acquisition,” Gambini says.
The team now hopes that their autoencoder will lead to new opportunities to examine organic materials and other delicate samples in unprecedented levels of detail, without the risk of damaging them.
The research is described in Machine Learning: Science and Technology.