While automation and machine-learning technologies hold great promise for radiation oncology programmes, speakers at the ASTRO Annual Meeting cautioned that significant challenges remain when it comes to clinical implementation. Joe McEntee reports
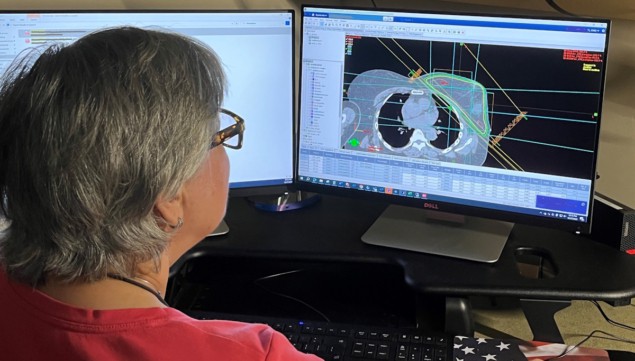
The automation of core processes in the radiation oncology workflow is accelerating, creating the conditions for technology innovation and clinical upside – at scale – across the planning, delivery and management of cancer treatment programmes. Think tumour and organ segmentation, optimized treatment planning, as well as a range of diverse tasks spanning treatment plan QA, machine QA and workflow management. The rulebooks, in every case, are being rewritten thanks to the enhanced efficiency, consistency and standardization promised by automation and machine-learning technologies.
That’s a broad canvas, but what about the operational detail – and workforce impacts – when deploying automation tools in the radiotherapy clinic? This was the headline question preoccupying speakers at a dedicated conference session – Challenges to Automation of Radiation Oncology Clinical Workflows – at the ASTRO Annual Meeting in San Diego, CA, earlier this month.
Zoom in to that radiotherapy workflow and the questions proliferate. Long term, what do the human–machine interactions look like versus the end-game of online adaptive radiotherapy tailored to the unique requirements of each patient? How will the roles of clinical team members evolve to support and manage increasing levels of automation? Finally, how do end-users manage the “black-box” nature of automation systems when it comes to the commissioning, validation and monitoring of new-look, streamlined treatment programmes?
Knowledge is power
When deploying automation and machine-learning tools in a radiotherapy setting, “we should have the right problem in mind – building things that are clinically relevant – and also have the right stakeholders in mind,” argued Tom Purdie, a staff medical physicist in the radiation medicine programme at Princess Margaret Cancer Centre in Toronto, Canada. At the same time, he noted, it’s vital to address workforce concerns about the perceived “loss of domain knowledge” that comes with the implementation of automation in the clinic, even when the end-user oversees and manages automated tools while still completing portions of the workflow that are yet to be automated.
As such, medical physicists and the wider cross-disciplinary care team will need to reimagine their roles to optimize their contribution in this “offline” mode. “So instead of looking at every patient and being able to deal with them,” Purdie added, “our contribution is going to be on how [machine-learning] models are built – to ensure there’s data governance, the right data’s going in, and that there’s data curation. This is the way to maintain our domain knowledge and still ensure quality and safety [for patients].”

Meanwhile, the technical and human factor-related challenges around adoption of automated treatment planning provided the narrative for David Wiant, a senior medical physicist at Cone Health, a not-for-profit healthcare network based in Greensboro, NC. The motivations for automated planning (AP) are clear enough – the relentless upward trajectory of cancer diagnoses in all the forecasts for the coming years. “It’s important that we treat these people as fast as we can,” Wiant told delegates.
The key to clinical success with AP lies in recognizing – and systematically addressing – the hurdles to its deployment. Workflow integration is a case in point. “A clinic needs to have a clear plan how to implement AP – who runs it, when it is used, on what cases,” Wiant noted. “If not, you can run into problems quickly.”
Then there’s reliability and the fact that AP can produce unexpected results. “There’ll be cases where you’ll put in what you think is a good, clean set of standard patient data and you’ll get a result you’re not expecting,” he continued. That’s almost always because the patient data have some unusual features – for example, implanted devices (or foreign objects) or perhaps a patient that’s undergone a previous course of radiation treatment.
The answer, posited Wiant, is to ensure the radiation oncology team has intimate knowledge of the AP to understand any reliability issues – and to use this knowledge to identify cases that need manual planning. At the same time, he concluded, “it’s important to identify sources of random error that may be unique to AP and add checks to mitigate [while] continuing to extend AP to handle non-standard cases.”
Guarding against complacency
Further downstream in the workflow, there are plenty of issues to consider with the roll-out of automated treatment planning QA, explained Elizabeth Covington, associate professor and director of quality and safety in the radiation oncology department at Michigan Medicine, University of Michigan (Ann Arbor, MI).
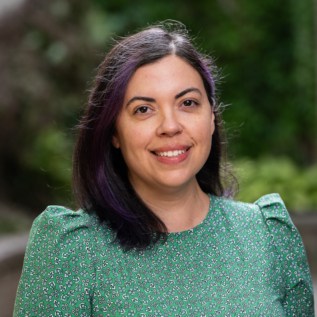
To avoid what Covington calls “imperfect automation” in treatment planning QA, it’s vital to understand the risk factors upfront, prior to implementation. Chief among these are automation complacency (the failure to be sufficiently vigilant in supervising automation systems) and automation bias (the tendency for end-users to favour automated decision-making systems over contradictory information, even if the latter is correct).
“It’s important when you start using these [automated plan QA] systems to understand the limitations,” said Covington. “[For example], you don’t want to release autochecks too soon that are going to give false-positives because users are going to get desensitized to the system flags.”
Granular software documentation is also mandatory, argues Covington. “Documentation is your friend,” she told delegates, “so that the whole team – physicists, dosimetrists, therapists – knows what these autochecks are doing and understands fully what the automation is telling them.”
The final “must-have” is prospective risk analysis of the automation software – whether it’s custom-built in-house code or a third-party product from a commercial vendor. “Before you release the software,” noted Covington, “you really need to understand what the risks and dangers are of integrating this software into your clinical workflow.”
Proton therapy on an upward trajectory while FLASH treatment schemes get ready to shine
With this in mind, Covington explained how she and her colleagues at Michigan Medicine quantify the risks of automation tools in terms of the so-called “software risk number” (SRN). The SRN is essentially a matrix of three discrete inputs: population (a direct measure of the patient population that the tool will impact); intent (how the software will be used in clinical decision-making and its ability to acutely impact patient outcomes); and complexity (a measure of how difficult it is for an independent reviewer to find an error in the software).
Covington concluded on a cautionary note: “For now, automation can solve some problems but not all problems. It can also cause new problems – issues you don’t anticipate.”