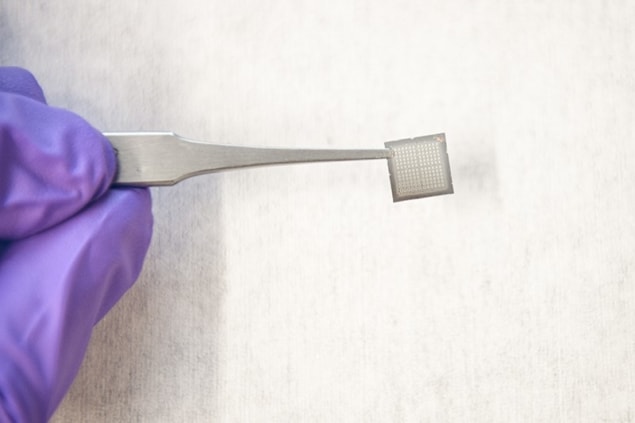
Researchers in the US have developed a perovskite-based device that could be used to create a high-plasticity architecture for artificial intelligence. The team, led by Shriram Ramanathan at Purdue University, has showed that the material’s electronic properties can be easily reconfigured, allowing the devices to function like artificial neurons and other components. Their results could lead to more flexible artificial-intelligence hardware that could learn much like the brain
Artificial intelligence systems can be trained to perform a task such as voice recognition using real-world data. Today this is usually done in software, which can adapt when additional training data are provided. However, machine learning systems that are based on hardware are much more efficient and researchers have already created electronic circuits that behave like artificial neurons and synapses.
However, unlike the circuits in our brains, these electronics are not able to reconfigure themselves when presented with new training information. What is needed is a system with high plasticity, which can alter its architecture to respond efficiently to new information.
Hydrogen doping
In their study, the team showed that this could be done with devices made from the perovskite crystal NdNiO3. They doped the material with hydrogen (proton) impurities, which increases local resistivity of the material. By applying single-shot electrical pulses across a device, they could shift the locations of the impurities, thereby changing the electronic properties of the device. A similar approach had been taken in other materials by moving around oxygen atoms, but this was much slower and less effective than moving the much smaller protons.
Electrically tuneable network learns fast
The team was able to to reconfigure their devices on demand, switching the function of a device between four options: resistor, memory capacitor, artificial neuron and artificial synapse. This reconfigurability is a first for a machine learning device. The team found that the device is very robust, retaining its functionality after being programmed over a million cycles. An additional benefit of the device is that it can be made using standard chip fabrication techniques.
To demonstrate its potential applicability to machine learning, the researchers did computer simulations of how the device would work in reconfigurable neural networks. The simulations suggest that the system would outperform static machine-learning architectures on two very important tasks. These are the recognition of numbers and letters from a large database of both handwritten and spoken digits; and the classification of heartbeat activity, as detected by an electrocardiogram.
The team is now developing large-scale test chips that it will use to build a computer inspired by the brain. The device is described in Science.