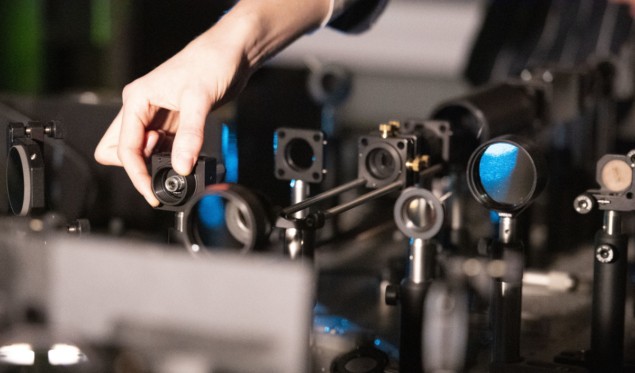
Fluorescence microscopy of live cells provides an indispensable tool for studying the dynamics of biological systems. But many biological processes – such as bacterial cell division and mitochondrial division, for example – occur sporadically, making them challenging to capture.
Continually imaging a sample at a high frame rate would ensure that when such divisions do occur, they will definitely be recorded. But excessive fluorescence imaging causes photobleaching and can prematurely destroy living samples. A slower frame rate, meanwhile, risks missing events-of-interest. What’s needed is a way to predict when an event is about to happen and then instruct the microscope to begin high-speed imaging.
Researchers at the Swiss Federal Institute of Technology Lausanne (EPFL) have created just such a system. The team developed an event-driven acquisition (EDA) framework that automates microscope control to image biological events in detail while limiting stress on the sample. Using neural networks to detect subtle precursors of events-of-interest, EDA adapts the acquisition parameters – such as imaging speed or measurement duration – in response.
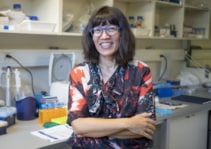
“An intelligent microscope is kind of like a self-driving car. It needs to process certain types of information, subtle patterns that it then responds to by changing its behaviour,” explains principal investigator Suliana Manley in a press statement. “By using a neural network, we can detect much more subtle events and use them to drive changes in acquisition speed.”
The EDA framework, described in Nature Methods, consists of a feedback loop between a live image stream and the microscope controls. The researchers used Micro-Manager software to capture images from the microscope and a neural network trained on labelled data to analyse them. For each image, the network output acts as a decision-making parameter to toggle between slow and fast imaging.
Event recognition
To demonstrate their new technique, Manley and colleagues integrated EDA into an instant structured illumination microscope and used it to capture super-resolved time-lapse movies of mitochondrial and bacterial divisions.
Mitochondrial division is unpredictable, typically occurring once every few minutes and lasting tens of seconds. To predict the onset of division, the team trained the neural network to detect constrictions, a change in mitochondrial shape that leads to division, combined with the presence of a protein called DRP1 that’s required for spontaneous divisions.
The neural network outputs a heat map of “event scores”, with higher values (when both constrictions and DRP1 levels are high) indicating locations within the image where division is more likely to occur. Once the event score exceeds a threshold value, the imaging speed increases to capture the division events in detail. Once the score reduces to a second threshold, the microscope switches to low-speed imaging to avoid exposing the sample to excessive light.
The researchers performed EDA on cells expressing mitochondrion-targeted fluorescent labels. During each EDA measurement, the network recognized precursors to bacterial division nine times on average. This toggled the imaging speed from slow (0.2 frames/s) to fast (3.8 frames/s) for an average of 10 s, resulting in fast imaging for 18% of frames. They note that many sites accumulated DRP1 but did not lead to division. These sites did not trigger the network, demonstrating its ability to discriminate events-of-interest.
For comparison, the team also collected images at constant slow and fast speeds. EDA caused less sample photobleaching than fixed-rate fast imaging, enabling longer observations of each sample and increasing the odds of capturing rare mitochondrial division events. In some cases, the sample recovered from photobleaching during the slow imaging phases, enabling a higher cumulative light dose.
While bleaching was higher with EDA than for constant slow imaging, many EDA sessions reached 10 min without degradation of sample health. The researchers also found that EDA better resolved the constrictions preceding division, as well as the progression of membrane states leading to fission, as captured by the bursts of fast images.
“The potential of intelligent microscopy includes measuring what standard acquisitions would miss,” Manley explains. “We capture more events, measure smaller constrictions, and can follow each division in greater detail.”
Detecting bacterial division
Next, the researchers used EDA to study cell division in the bacteria C. crescentus. The bacterial cell cycle occurs on the timescale of tens of minutes, creating distinct challenges for live-cell microscopy. They collected data at a slow imaging speed of 6.7 frames/hr, a fast imaging speed of 20 frames/hr or a variable speed switched by EDA.
The team found that the event-detection network developed for mitochondrial constrictions could recognize the final stages of bacterial division without additional training – likely due to similarities in constriction shape and the presence of a functionally similar molecular marker.
Improved microscopy technique sees living cells with seven times more sensitivity
Again, EDA reduced photobleaching compared with constant fast imaging, and measured constrictions with significantly smaller average diameters than with constant slow imaging. EDA enabled imaging of the entire cell cycle and provided details of bacterial cell division that are difficult to capture using a fixed imaging speed.
Manley tells Physics World that the team also plans to train neural networks to detect different kinds of events and use these to evoke different hardware responses. “For example, we envision harnessing optogenetic perturbations to modulate transcription at key moments in cell differentiation,” she explains. “We also think of using event detection as a means of data compression, selecting for storage or analysis the pieces of data that are most relevant to a given study.”
- To enable researchers to implement EDA on a wide variety of microscopes, the team is providing the control framework as an open-source plug-in for the Micro-Manager software.