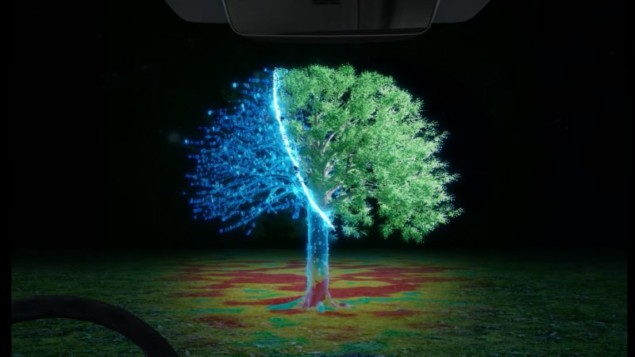
A thermal imaging system that uses machine learning to disentangle the information contained in infrared images has been unveiled by researchers at Purdue University in the US. Dubbed HADAR, the system could allow passive thermal imagers to create images that appear as if they were taken in broad daylight – according Zubin Jacob and colleagues.
Our ability to detect and classify images in low-visibility and night-time conditions has been transformed by technologies such as sonar, radar and LiDAR. These systems involve sending out a signal (sound, radio, light etc.) signal and detecting reflections. However, this makes it difficult for multiple versions of the same system to be used in close proximity without interference occurring. This makes these technologies unsuitable for some emerging technologies like self-driving vehicles.
Thermal imaging offers a possible solution to this problem, since it can passively observe night-time scenes using the infrared radiation emitted by objects. However, images taken by conventional infrared cameras tend not to have fine features and instead appear blurry. This makes such cameras unsuitable as replacements for technologies like LiDAR.
Scattered radiation
“The key reason for ‘blurry’ thermal images is that thermal imaging collects both direct emission of the targets and the scattered thermal radiation of other environmental objects,” Jacob explains. “Direct emission is usually ten times stronger than the scattered signal, but the former is textureless while the latter carries the textures.”
As an example of this effect in the visible range, picture a lightbulb. While the bright light it emits when turned on does not contain any discernible details of textures on the bulb’s surface, these details will appear when the bulb is illuminated by another light source.
In their study, Jacob’s team developed a far more advanced approach to thermal imaging. Called heat-assisted detection and ranging or HADAR, it is precise enough to pick up geometric textures in weak, scattered infrared signals.
Hyperspectral imaging
“We achieve this goal using thermal physics and machine learning, combined with spectral resolution in thermal images,” Jacob explains. “HADAR uses hyperspectral thermal imaging which takes thermal images of the scene for hundreds of different colours in the thermal infrared.”
In our own eyes, colours in the visible spectrum are processed by a combination of red, green, and blue photoreceptors. In comparison, HADAR builds up infrared images based on three key attributes of the objects it observes. These are an object’s temperature (T); emissivity (e) – which varies with material composition; and texture (X), which generates unique patterns of thermal radiation.
All of these values can be gleaned from the infrared light emitted by a scene, but are initially mixed together in the cluttered raw data. But using machine learning, the team’s “TeX vision” approach can disentangle the three key attributes to recover the weak geometric features in infrared signals that are typically blurred out.
TeX colours
This is done using an algorithm that assigns different “colours” to different parts of the scene. Since different materials can be identified by a combination of their T, e, and X values, Jacob’s team could build up a semantic library of suitable colours.
“The semantic library comes with a colour for each semantic label, for example, blue for water, green for tree, and yellow for sand,” Jacob explains. “Colours for materials are purely determined according to their daily visual appearance, to mimic daylight optical imaging.” With this approach, HADAR could image low-visibility and night-time scenes as they appear in broad daylight.
Single-photon LIDAR system images 3D objects underwater
The researchers acknowledge there is still some way to go before HADAR becomes widely available, especially since the hyperspectral thermal imagers currently available are bulky, slow, and expensive. Yet through further research, they are hopeful that these challenges could be addressed in the next few years – leading to high-speed, compact, and lightweight thermal imagers for HADAR.
If achieved, the technology could open up new opportunities in a wide array of useful applications. “We think HADAR can be useful for autonomous navigation, robotics, smart healthcare monitoring, especially at night,” says Jacob. “Many wild animals are only active at night where regular cameras do not work, we think HADAR may be useful for wildlife monitoring as well.”
The HADAR system is described in Nature.