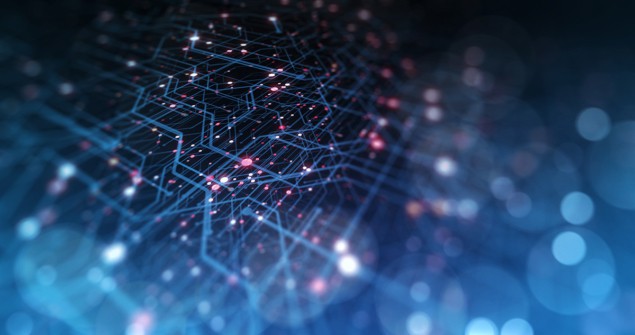
Two researchers in China have shown how unwanted quantum tunnelling in field-effect transistors (FETs) could be suppressed by controlling the lattice orientations of materials used in the devices. Using machine learning to analyse thousands of candidate orientations, Ye-Fei Li and Zhi-Pan Liu at Fudan University in Shanghai identified two stable configurations that minimize tunnelling. Their research could allow further device miniaturization, which is limited by the negative effects of tunnelling.
FETs are key components of most modern computers and electronics. In many existing designs, a silicon semiconductor channel is covered by insulating silicon dioxide and then by a gate electrode. The channel’s conductivity is controlled by the gate electrode, which applies a voltage perpendicular to the current flow through the channel. By varying the voltage, the current in the channel can be switched on and off.
As manufacturing techniques have improved, FETs have steadily reduced in size. This is famously described by Moore’s law, which says that the number of transistors that can fit on a computer chip doubles roughly every two years. However, as FETs approach nanometre channel lengths, quantum physics looks set to wreak havoc with further miniaturization.
Tunnelling carriers
One problem is that the insulating layer that separates the gate from the channel will become so thin that charge carriers can quantum mechanically tunnel between the gate and channel – thwarting the FET’s operation. So minimizing tunnelling at this interface will play an important role in further miniaturization.
Silicon and silicon dioxide have different crystal structures. This means that atoms at the interface between the two materials can adopt a range of different structures depending on the relative orientation of the silicon and silicon dioxide crystals. Some of these interface structures will encourage tunnelling, while others will suppress it.
Negative capacitance could make transistors more energy efficient
In their study, Li and Liu examined how tunnelling is affected by interface structure. Using machine learning, they generated close to 2500 possible structures, and assessed how appropriate they would be for use in FETs. They found that only 40 configurations repeated themselves every nanometre, which was their target length for a channel. Of these, only 10 structures were energetically stable. When the ability to suppress tunnelling was considered, only two candidate structures remained.
The duo hopes that their findings will allow engineers to further shrink FETs, while minimizing the effects of tunnelling. They also point out that their approach is general and can be applied to materials beyond silicon and silicon dioxide so it could help improve the designs of transistors made of other semiconductors such as gallium nitride and silicon carbide.
The research is reported in Physical Review Letters.