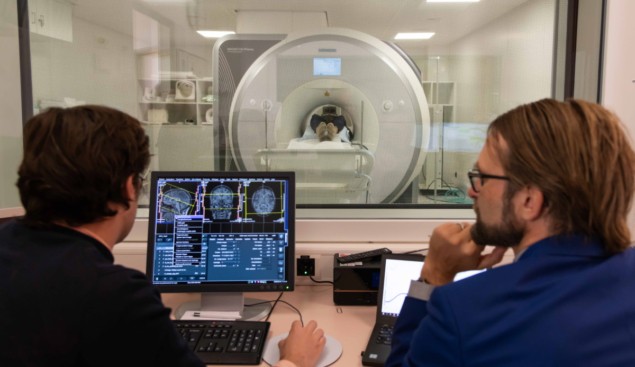
Stroke – a life-threatening condition arising from restriction of the blood supply to the brain – is a leading cause of long-term disability. In Europe, there are over 1.5 million new cases each year, with less than 15% of patients achieving full recovery. A key challenge in stroke rehabilitation is that recovery varies widely between patients: roughly two thirds of stroke patients recover naturally from their initial impairments, the remainder do not. Critically, natural recovery is especially variable in severely impaired patients.
The ability to forecast an individual’s potential recovery could help doctors select personalized therapy and maximize treatment outcome, particularly for those with severe impairments. With this aim, an international research team headed up at the Swiss Federal Institute of Technology (EPFL) has developed a machine-learning system that makes high-accuracy predictions of recovery outcome for stroke patients.
“The key is to find the optimal neuro-rehabilitative strategy to maximize individual treatment outcome,” explains EPFL’s Friedhelm Hummel in a press statement. “This tool can support the prediction of individual courses of recovery early on and will have an important impact on clinical management, translational research and treatment choice.”
Personalized predictions
Hummel and collaborators developed the predictive tool based on analysis of the connectome – a map of the whole brain’s wiring generated from multiple MR images. Their goal was to use information from whole-brain structural connectomes as a potential prognostic factor to determine individual outcome after stroke (recovery versus no recovery), using a machine learning approach based on support vector machines (SVMs).
To train their prediction model, the researchers analysed whole-brain structural connectomes of 63 patients two weeks after stroke onset, while simultaneously assessing the patients’ motor impairment. Within this dataset, 39.7% of the patients did not show natural recovery; in a subgroup with severe motor impairment, 63.9% did not naturally recover. The team also tracked connection changes in the patients’ brains up to three months later.
The SVMs were trained to separate patients who recovered naturally from those who did not, based on their connectomes. The SVMs then defined the underlying brain network patterns for each patient to make predictions about their recovery potential, with a particular focus on those who were severely impaired.
Internal validation of the prediction model revealed that features extracted from the connectomes two weeks after stroke could segregate patients with recovery from those without. Internal validation of the model demonstrated high classification performance, with a prediction accuracy of 83% for all patients. Importantly, the classification accuracy was even higher – 92% – in the difficult-to-predict group of severely impaired patients.

The researchers further validated the results in a smaller independent dataset. Here, the classification across all subjects, including severely impaired patients, also achieved high accuracy. They note, however, that these results should be taken with caution, as the external validation data sample was small.
The areas that matter
The team also used the whole-brain connectomes to identify relevant brain areas and connections that may support or hamper motor recovery, determining network nodes of particular importance for recovery in severely impaired patients. The identified brain areas are likely to underlie the processes of spontaneous biological recovery of motor functions and could influence the design of new therapies.
Feature extraction revealed that connectivity within the core parietofrontal motor network is crucial for favourable outcomes, with secondary motor areas especially important in patients with severe initial impairment. In addition, functional systems such as the attentional, somatosensory or multimodal areas clearly contribute to motor function recovery and improve the classification.
One image, one prediction: MRI may foretell cognitive decline after stroke
The researchers, who report their findings in Brain, conclude that computational analyses of whole-brain connectomes have high potential for predicting patients’ degree and course of recovery at an early stage following stroke. Hummel notes that for routine clinical use, the prediction tool will require further development and, ideally, automation.
“The next steps we are planning are to further evaluate this approach in other data sets and develop the analytical approaches towards a simpler application for direct clinical use,” he tells Physics World. Furthermore, we continue to analyse structural and functional connectomics and its impact on stroke recovery to use this information towards personalized treatment strategies.”